Quantitative Momentum Strategies: Capturing Alpha through Systematic Trend-Following Techniques
Momentum trading has been a staple of financial markets for decades, offering traders a data-driven method to capitalise on price trends. Quantitative momentum strategies have taken this approach to the next level, using systematic, algorithmic techniques to enhance consistency and reduce human bias. The ultimate goal of these strategies is to capture alpha — the excess return beyond a benchmark — by following well-defined trends in the market. In this article, we explore how quantitative momentum strategies work, their core components, and how traders can use them to achieve better performance.
Understanding Momentum Trading
Momentum trading is based on a simple principle: assets that have performed well recently will likely continue to do so in the short term, while poorly performing assets will likely continue their downward trajectory. This is often described as “riding the wave” — buying into uptrends and selling into downtrends. For traders looking to apply momentum strategies, especially those interested in international markets, the opportunity to trade in US stocks is significant. The US stock market, with its high liquidity and diverse asset classes, is a prime arena for momentum trading.
The historical roots of momentum trading can be traced back to various academic studies, one of the most influential being the research by Jegadeesh and Titman in 1993, which demonstrated the efficacy of momentum strategies across asset classes. Their findings showed that stocks with strong past performance over three to twelve months continued to outperform, while those with poor past performance lagged. This created the foundation for modern momentum strategies, which traders have since refined using quantitative techniques.
Momentum strategies generally fall into two categories: cross-sectional momentum, where traders compare the performance of various assets relative to each other, and time-series momentum, where traders focus on the individual performance of an asset over time. Both approaches rely heavily on the key concepts of trend, volatility, and price persistence. Understanding these underlying forces helps traders make sense of the market dynamics and develop robust strategies.
Introduction to Quantitative Momentum Strategies
Quantitative strategies use statistical models and data analysis to remove emotions and subjective decisions from trading. This makes the approach more consistent and reliable, as decisions are based on cold, hard data rather than instinct. The goal is to identify trends earlier and more accurately than traditional discretionary trading methods.
Momentum lends itself well to quantitative strategies because trends can be measured and analysed systematically. By quantifying momentum, traders can set clear, rule-based criteria for entering and exiting trades. The most popular momentum models include simple moving averages (SMA), exponential moving averages (EMA), and the relative strength index (RSI). These indicators provide objective signals on whether an asset is in an uptrend or downtrend, allowing traders to act accordingly.
For example, a simple moving average strategy may involve buying a stock when its short-term moving average crosses above its long-term moving average, signalling the beginning of a new uptrend. Similarly, the RSI can be used to measure the strength of price movement, helping traders identify when an asset is overbought or oversold.
Core Components of Systematic Trend-Following Techniques
A well-designed quantitative momentum strategy relies on several core components. The first is trend detection algorithms. These algorithms are responsible for identifying price trends and determining when they are strong enough to warrant a trade. Algorithms often use technical indicators such as moving averages, RSI, or MACD to assess trends. Once a trend is identified, the system signals a buy or sell order, depending on the direction of the trend.
Risk management is another critical element. Momentum trading can be risky, particularly if a trend reverses unexpectedly. A robust strategy will include stop-loss orders and position-sizing techniques to limit potential losses. Position sizing ensures that a trader does not overexpose themselves to any single trade, while stop-loss orders automatically sell an asset if it drops below a certain price, preventing further losses.
Implementing Quantitative Momentum Strategies
Implementing a quantitative momentum strategy involves a series of steps. First, traders must define their universe of assets. This could range from stocks and bonds to commodities and cryptocurrencies, depending on the trader’s expertise and goals. Next, they set parameters for trend detection, such as the length of moving averages or the levels of the RSI that indicate overbought or oversold conditions.
Once the strategy parameters are in place, traders must establish risk management protocols. This includes determining the size of each trade relative to their total portfolio and setting stop-loss levels to protect against large losses. For those using automated systems, trade execution can be handled programmatically, ensuring that trades are executed as soon as the predetermined conditions are met.
Data inputs are critical to momentum strategies. Traders rely on price data, volume data, and volatility metrics to inform their algorithms. More advanced strategies may also incorporate alternative data sources, such as social sentiment or macroeconomic indicators, to gain a broader perspective on market trends.
Conclusion
Quantitative momentum strategies offer a powerful way for traders to capture alpha by systematically following trends. By combining trend detection algorithms, risk management protocols, and robust backtesting techniques, traders can create a reliable and scalable approach to trading. However, like any strategy, momentum trading comes with risks, including trend reversals and overfitting.
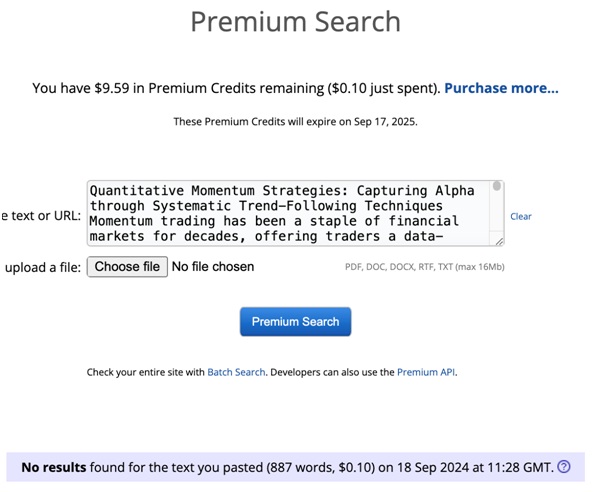
Comments are closed.